Tuomas Oikarinen
UC San Diego
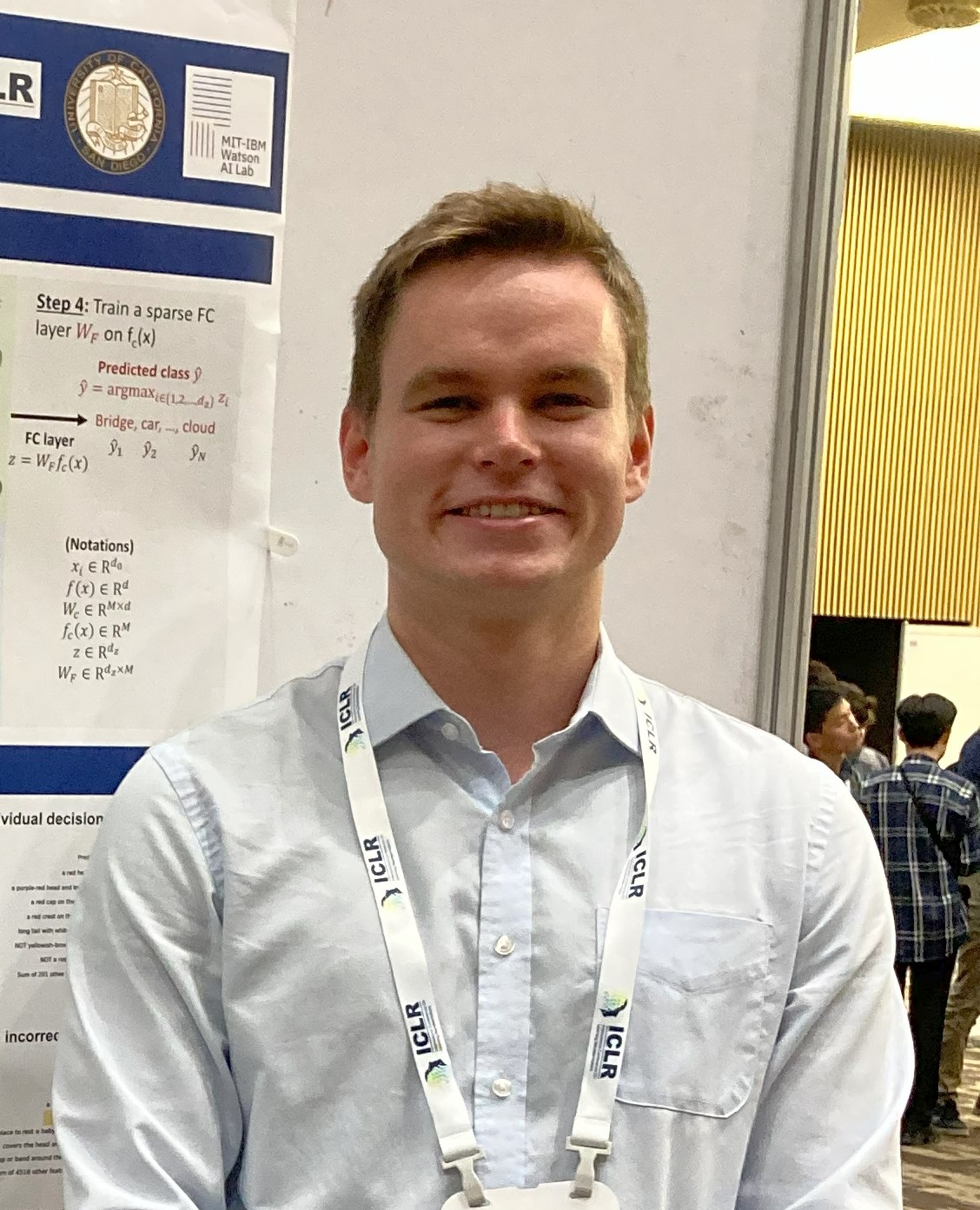
Developing scalable ways to understand deep learning. Especially excited about using (mechanistic) interpretability to help improve safety and reliability of neural networks.
PhD student at UC San Diego advised by Prof. Tsui-Wei (Lily) Weng. Undergrad from MIT.
Google Scholar / Github / email: toikarinen@ucsd.edu
news
Oct 27, 2023 | Our paper The Importance of Prompt Tuning for Automated Neuron Explanations was accepted to NeurIPS 2023 workshop ATTRIB! This paper shows that simple prompt tuning can significantly increase efficiency and accuracy of automated explanations of neurons in LLMs. |
---|---|
Jul 13, 2023 | Our paper Corrupting Neuron Explanations of Deep Visual Features led by Divyansh Srivastava was accepted at ICCV 2023! This paper highlights the brittleness of Neuron-level Interpretability Methods. |
Jan 20, 2023 | Two papers accepted to ICLR 2023! CLIP-Dissect(Spotlight) presents a new efficient and automated way to describe hidden layer neurons in vision models. Label-Free Concept Bottleneck Models proposes a way to turn existing black-box models into interpretable Concept Bottleneck Models where each neuron corresponds to a human understandable concept. |